Artificial intelligence (AI) is no longer a future-facing concept in manufacturing. It is a present-day force accelerating productivity, precision, and decision-making across the industrial sector.
As manufacturers adopt AI-driven systems to optimize operations and reduce downtime, these technologies are becoming central to the evolution of Industry 4.0, where data and automation reshape how products are made and maintained. This guide by 10X Engineered Materials covers use cases, benefits and the future of AI in manufacturing.
Applications of AI in Manufacturing
By integrating machine learning algorithms and vision systems into operations, companies can unlock new levels of precision and efficiency previously unattainable.
AI-powered vision systems use high-resolution cameras and deep learning models to perform real-time inspections of components and finished products. They are capable of detecting surface defects, dimensional inaccuracies, and pattern deviations with greater speed and consistency than human inspectors. Automation benefits include:
- A reduction in subjectivity.
- Shortened cycle times.
- Closed-loop feedback systems.
These closed-loop feedback systems are where process parameters adjust automatically in response to detected variations, contributing to higher product consistency and lower defect rates.
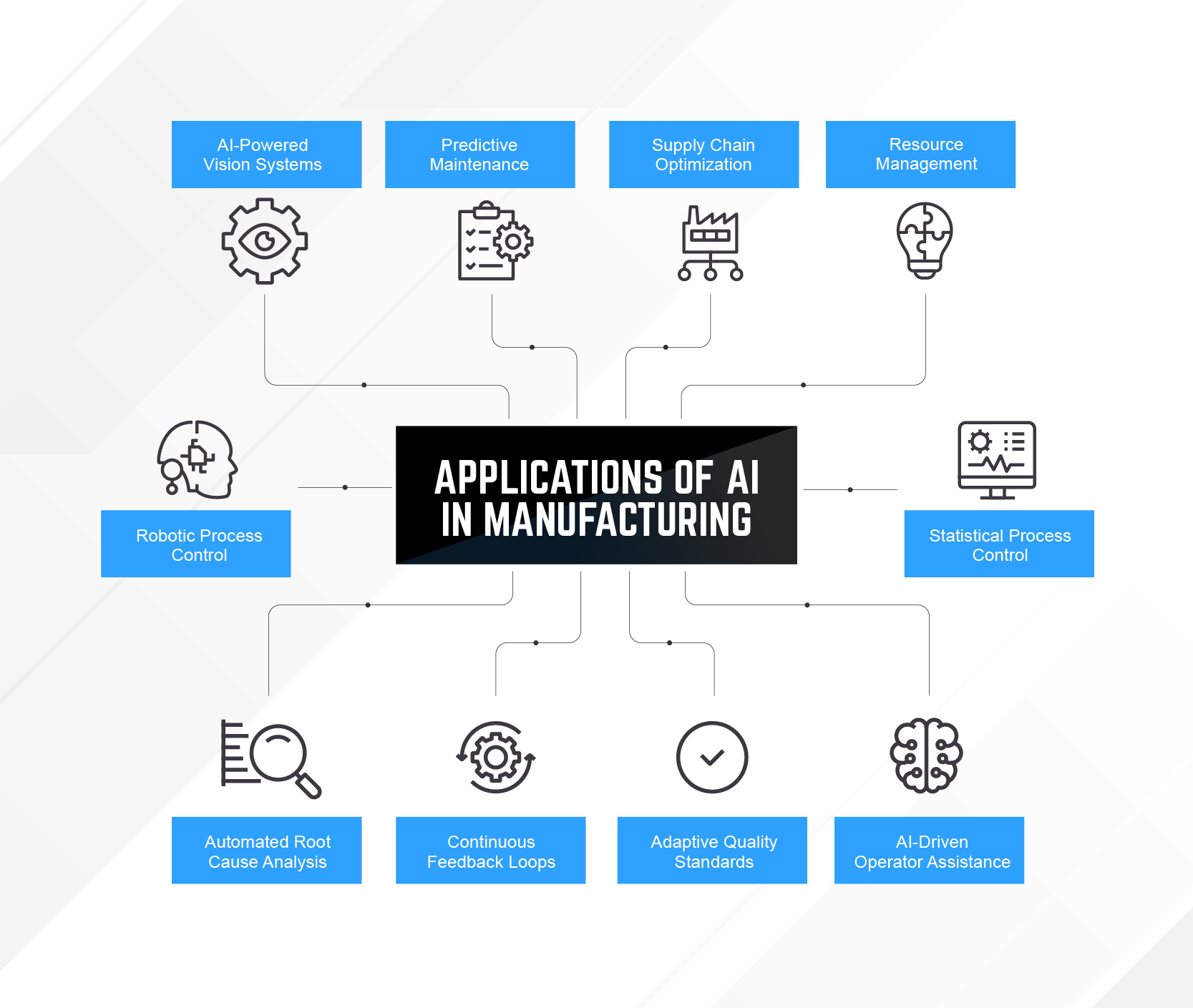
Predictive Maintenance
Machine-learning algorithms analyze time-series sensor data like vibration, temperature, pressure, and acoustic signals from production equipment to identify early indicators of wear and tear. These capabilities allow maintenance teams to service equipment proactively instead of reactively, which increases equipment uptime by 20%.
In abrasive blasting operations, where components like nozzles, hoses, and compressors degrade with use, predictive models can anticipate when performance thresholds are nearing critical limits. This knowledge can reduce system downtime by 35%, lower maintenance costs by 28%, and provide an additional layer of worker safety.
Supply Chain Optimization
Supply chain operations benefit significantly from AI’s ability to analyze large volumes of structured and unstructured data in real time. Applications include:
- Demand forecasting using historical sales data and external variables like market trends.
- Inventory optimization to reduce excess stock while minimizing stockouts.
- Dynamic pricing and procurement models that adjust sourcing decisions based on supplier performance, availability, and cost fluctuations.
In sectors like surface preparation, where abrasive materials must be restocked with high precision to match job-specific needs, AI can help forecast usage patterns and automate reorder points to improve material availability and reduce waste.
How AI Is Enhancing Efficiency and Productivity
AI applications drive physical actions through robotics, optimize workflow with intelligent decision-making, and help manufacturers better manage materials, energy, and labor.
For example, Siemens is introducing enhancements in their Industrial Copilot that will yield up to a 50% increase in productivity. Another example is the recent launch of Spark.DT, a collaboration between 10X Engineered Materials and TeamAI. The system delivers real-time, context-aware guidance for abrasive blasting. Site visitors can use this AI tool to access quick information on abrasive selection, equipment setup, safety practices, and operational efficiency from plain-language queries.
In the manufacturing process itself, AI-enabled robotic algorithms in abrasive blasting can dynamically adjust nozzle angles, standoff distances, and traverse speeds based on surface geometry and material condition. The result is more uniform surface finishes and minimized abrasive use — two critical factors for quality and cost efficiency in aerospace, automotive, and heavy equipment maintenance industries.
Key productivity benefits of AI in manufacturing include:
- Adaptive control, where robots respond to changes in part orientation, size, and defects.
- Collaborative automation, where collaborative robots, or cobots, work alongside humans, sharing tasks safely and efficiently.
- Reduced cycle times, where automated systems operate with consistent speed and accuracy, help meet or exceed Takt Time.
Process Optimization
AI supports continuous improvement in manufacturing by identifying inefficiencies and recommending or executing process changes. Machine learning models trained on historical production data can pinpoint bottlenecks, predict quality deviations, and suggest optimal parameter settings.
Consider a high-throughput line where abrasive blasting is one of several value-added steps. AI can optimize upstream and downstream processes to synchronize with blasting throughput, ensuring balanced workloads and minimal idle time. It can also correlate variables such as abrasive type, pressure, and humidity to final finish quality, recommending adjustments to maximize yield.
Techniques used include:
- Reinforcement learning for iterative optimization of process parameters.
- Digital twins to simulate and refine production scenarios in a virtual environment.
- Constraint-based scheduling for real-time production planning.
Resource Management
AI is instrumental in managing finite resources, whether it is raw materials, energy, water, or labor. These systems analyze usage patterns, forecast demand, and recommend adjustments to reduce waste and costs while maintaining throughput. Across broader manufacturing operations, AI improves resource efficiency by:
- Adjusting energy-intensive processes to align with off-peak utility rates or renewable energy availability.
- Using scheduling tools to match workforce availability to production demand as needed.
- Reducing overstocking and shortages while maintaining service levels.
How AI Is Improving Quality Control and Reducing Defects
Rather than simply flagging deviations, AI systems proactively learn from production data to prevent defects before they occur.
Advanced machine learning models can be trained on historical production data to recognize normal patterns of variation. When new data deviates from expected behavior, whether from equipment sensors, visual inspection systems, or material characteristics, the system flags it immediately. These visual inspection systems may reduce defects by up to 90% when compared to manual methods.
Statistical Process Control
Traditional statistical process control (SPC) relies on predefined limits and assumes normal data distribution. AI-based systems go further by continuously learning from live process inputs and adapting thresholds dynamically. This level of understanding allows them to identify subtle process shifts and nonlinear relationships that SPC might overlook.
AI can also account for multivariate influences, such as the combined effects of humidity, machine wear, and operator behavior. Cumulatively, this information gives stakeholders a more holistic view of the process and the root causes of defects.
Automated Root Cause Analysis
AI speeds up root cause analysis through pattern recognition and correlation analysis. Instead of manually reviewing process logs or inspection reports, AI systems can comb through vast datasets to pinpoint causal relationships between inputs and defects.
Continuous Feedback Loops
AI also facilitates closed-loop quality control structures that continuously adjust process parameters based on inspection outcomes. Vision systems and sensors feed data into the model, which evaluates product quality and automatically updates equipment settings to maintain optimal performance. This approach supports zero-defect manufacturing, where quality is worked into the process and maintained without constant human oversight.
Adaptive Quality Standards
In high-mix or custom manufacturing environments such as parts production for aerospace, medical devices, or energy infrastructure, AI can dynamically adjust quality criteria based on:
- Specific tolerances.
- Material grades.
- Customer requirements.
This capability ensures the inspection system adapts to varied conditions without manual reconfiguration.
How Abrasive Blasting Can Improve With AI
While abrasive blasting is a mature and mechanically driven process, AI introduces new capabilities that can significantly improve precision, repeatability, safety, and traceability. These advancements go beyond basic automation, enabling smarter, data-informed operations that adapt quickly and optimize for both performance and cost.
With AI integration, abrasive blasting improves through:
- Equipment health: AI-enabled systems reduce manual labor in hazardous tasks like abrasive blasting processes. Operators using robotic systems with abrasive media that generate less airborne particulate reinforce AI safety benefits. This addition aligns with predictive health monitoring technologies that AI platforms support.
- Environmental compensation: Environmental conditions can affect abrasive blasting performance. AI models can account for these variables, make dynamic adjustments, and improve reliability in outdoor or mobile blasting operations where conditions are less controlled.
- Process parameter optimization: AI systems use historical data to fine-tune blast parameters like media flow rate, pressure, stand-off distance, and nozzle dwell time. This optimization ensures a consistent surface roughness and minimizes over-blasting, which can increase finishing costs and weaken substrates.
- Digital traceability: AI can support automated documentation for each blasting cycle, capturing data on part ID, media usage, blast time, and process conditions. This data can be used for quality audits, compliance with surface preparation standards such as SSPC SP-10, and customer reporting.
- Operator assistance: AI-powered interfaces can assist operators by providing feedback on technique, using motion tracking and camera inputs. In training, AI-driven simulators can replicate blasting conditions and give instant feedback.
- Integration with robotics: AI enables adaptive path planning for irregular or complex surfaces. It generates optimized blasting trajectories, ensuring uniform coverage and reducing masking errors.
The Future of AI in Manufacturing
AI is becoming deeply embedded in every layer of manufacturing. Experts predict that AI in manufacturing will reach a market size of $20.8 billion by 2028, with a compound annual growth rate of 45.6% between 2023 and 2028.
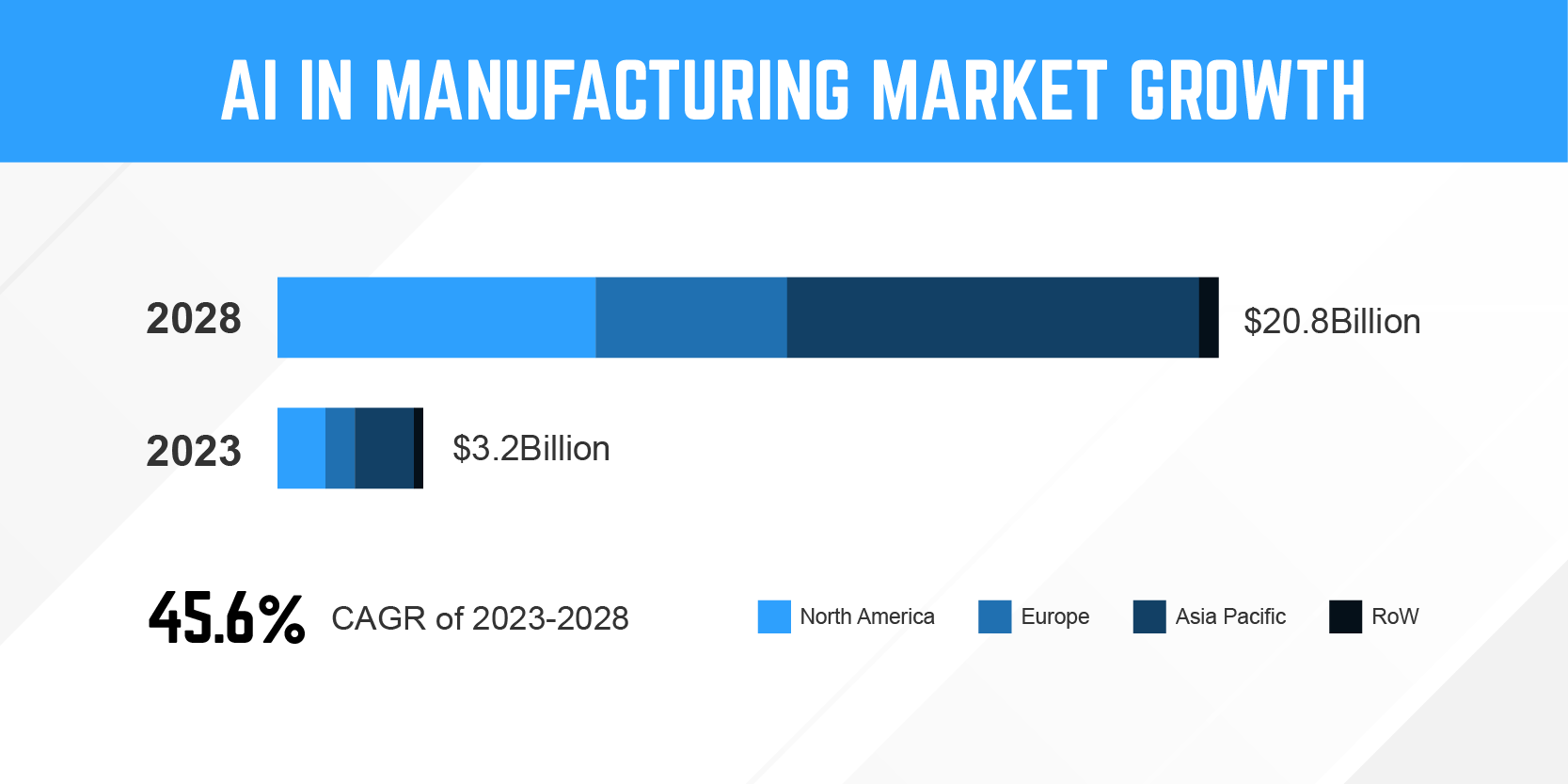
This shift will transform how factories operate and redefine the roles of people, systems, and key infrastructure. Industries will see a significant increase in quality control, supply chain optimization, and predictive maintenance. Three key technology trends to keep an eye on include:
1. Edge Computing
As manufacturers generate more data from sensors, machines, and vision systems, edge computing will play a central role in AI deployment. Edge computing processes data locally at or near the source, enabling ultra-low latency decisions on the factory floor. Applications include:
- Instant equipment control and failure detection.
- On-the-fly adjustments during production.
- Faster response times for robotics and machine vision.
2. Digital Twins
Digital twins, which are virtual replicas of physical assets, will become standard tools for modeling, simulating, and optimizing production environments. These systems integrate fresh data from sensors and AI algorithms to provide a live operational model of machines, processes, or entire facilities. Manufacturers will increasingly use digital twins to:
- Simulate production changes before deployment.
- Test maintenance strategies and system configurations.
- Optimize energy and material usage.
- Predict how variables affect outcomes in complex processes like surface preparation or precision machining.
3. Human-AI Collaboration
Rather than replacing workers, AI will cement itself as a decision-support tool like Spark.DT, to guide operators, engineers, and managers through complex tasks. This human-AI collaboration will be central to the success of intelligent factories. AI assistants will become common practice in providing:
- Recommendations on process improvements or machine settings.
- Predictive alerts with clear explanations of risk and action.
- Context-aware insights based on historical and real-time data.
- Voice-controlled and gesture-controlled interfaces for hands-free assistance.
Assessing AI Readiness in Manufacturing
To thrive in an AI-enabled landscape, the workforce needs to evolve alongside the technology. Operators, technicians, and managers must understand how to interpret and act on AI data, requiring a basic statistical understanding, familiarity with dashboards, and the ability to question model outputs.
As AI integrates into machines and control panels, workers will learn to operate and troubleshoot systems using new interfaces like augmented reality, natural learning commands, and AI-driven human-machine interfaces.
For organizations considering AI integration, a structured framework helps evaluate readiness and identify viable implementation areas:
- Data availability: Assess whether operational data is being collected, stored, and structured in ways AI models can use. Sensorization and digital instrumentation are top prerequisites.
- Process stability: AI models perform best in environments with consistent process variables. Evaluate whether current workflows are repeatable and well-documented.
- Workforce readiness: Invest in upskilling teams to work alongside AI tools. Technical staff should understand how to interpret AI outputs and integrate them into decision-making.
- Use case identification: Prioritize applications with a high potential return on investment, like quality inspection, predictive maintenance, or supply chain optimization.
- Technology infrastructure: Ensure the facility has the IT architecture to support AI deployment. Examples include cloud platforms, edge computing devices, and secure data pipelines.
AI Integration is at the Forefront of Modern Manufacturing
As AI solutions gain traction in manufacturing, they signal a broader movement — one in which intelligent tools amplify human expertise, making specialized knowledge more accessible and operations more efficient. From automating quality control and predicting equipment failure to optimizing supply chains and enabling intelligent surface preparation through tools like Spark.DT, AI is ushering in a new era of precision, efficiency, and adaptability.
Success in this evolving landscape depends on more than technology. It requires a workforce equipped with data literacy, cross-disciplinary skills, and a commitment to continuous learning. It calls for deliberate strategy, scalable infrastructure, and thoughtful implementation. For those ready to embrace it, AI offers a path toward more resilient, sustainable, and future-ready manufacturing.